Building the best model of brain activity: We're Leading The Neural Latents Benchmark Challenge
"We don't quit at halftime" (But we are in the lead)
From returning agency to a human being with paralyzed limbs, to restoring auditory function, to treating depression, the concept of a brain-computer-interface (BCI) presents a mind-boggling set of possibilities. One day, rather than pounding keyboards and sliding mice to convey our intentions to our laptops, our thoughts will simply elicit the desired responses. Perfect transcription fluency1 would revolutionize how human beings invest their professional and leisure hours and redefine the term “able-bodied.”
Realizing the promise of BCI means understanding the activity of the 80 billion neurons firing in our brains, then translating the complexity of that activity into “latent” patterns. For instance, while we speak to one another, you have no idea whatsoever about the specific neurons firing in my brain, but you can discern a “latent” pattern, like the nodding of my head in agreement when you describe how a Bruce Springsteen concert is a religious experience or the visceral, snarling anger on my face when you tell me you root for the Dallas Cowboys.
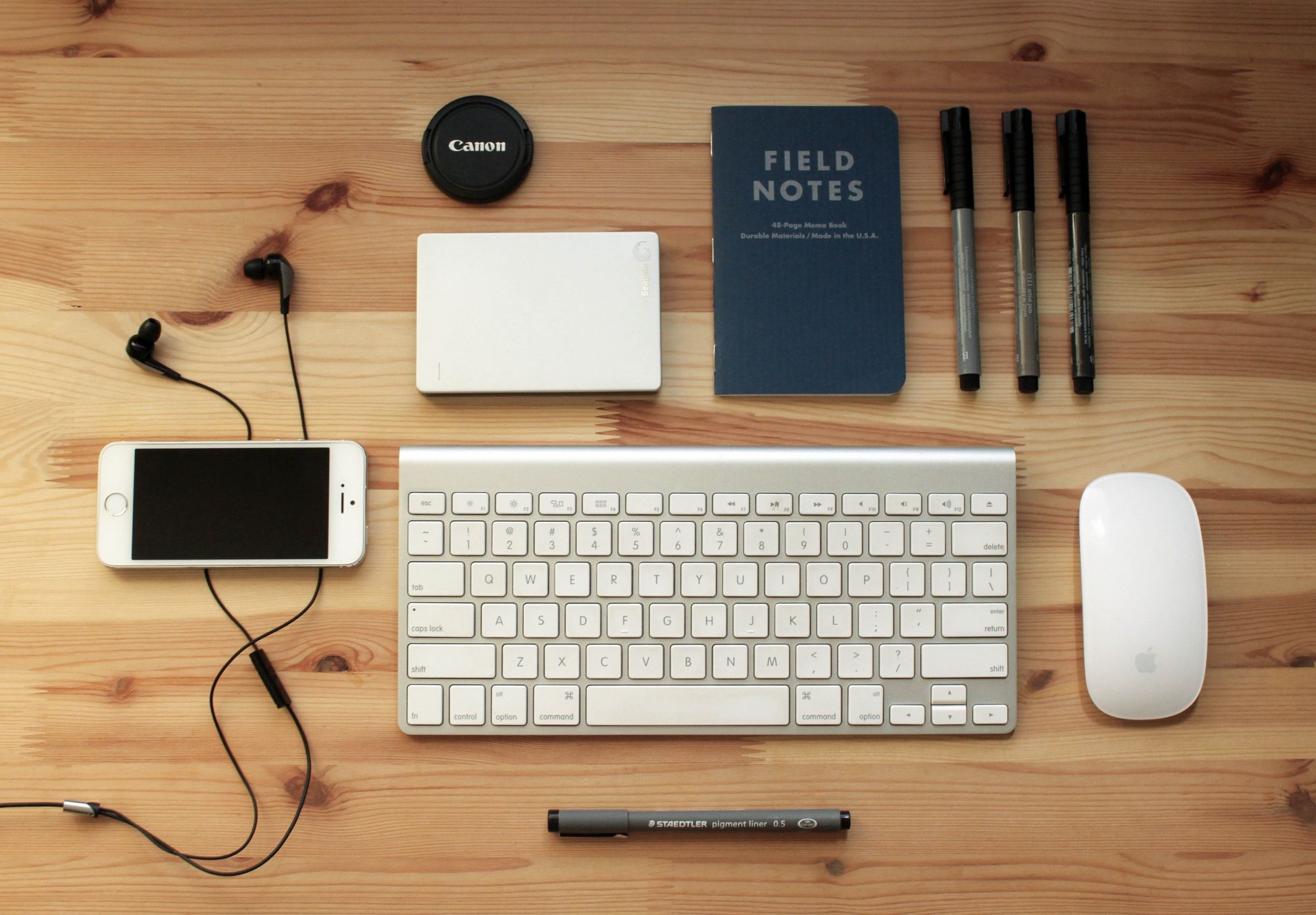
On September 5th, 2021, while most of us were fixated on the beginning of the upcoming football season, a less publicized, but perhaps more important competition began. The Neural Latents Benchmark Challenge begins with data from (primarily) Utah Arrays implanted in monkey brains. Electrodes read the behavior of 100+ monkey neurons. The mathematical challenge is simply stated: determine if an additional few dozen neurons are active or not. As an analogy, consider an enormous checkerboard grid. Now remove the colors. The grid contains 80 billion squares. You are told the color (red or black) of ~100 squares. You are instructed to determine the color (red or black) of an additional few dozen squares.
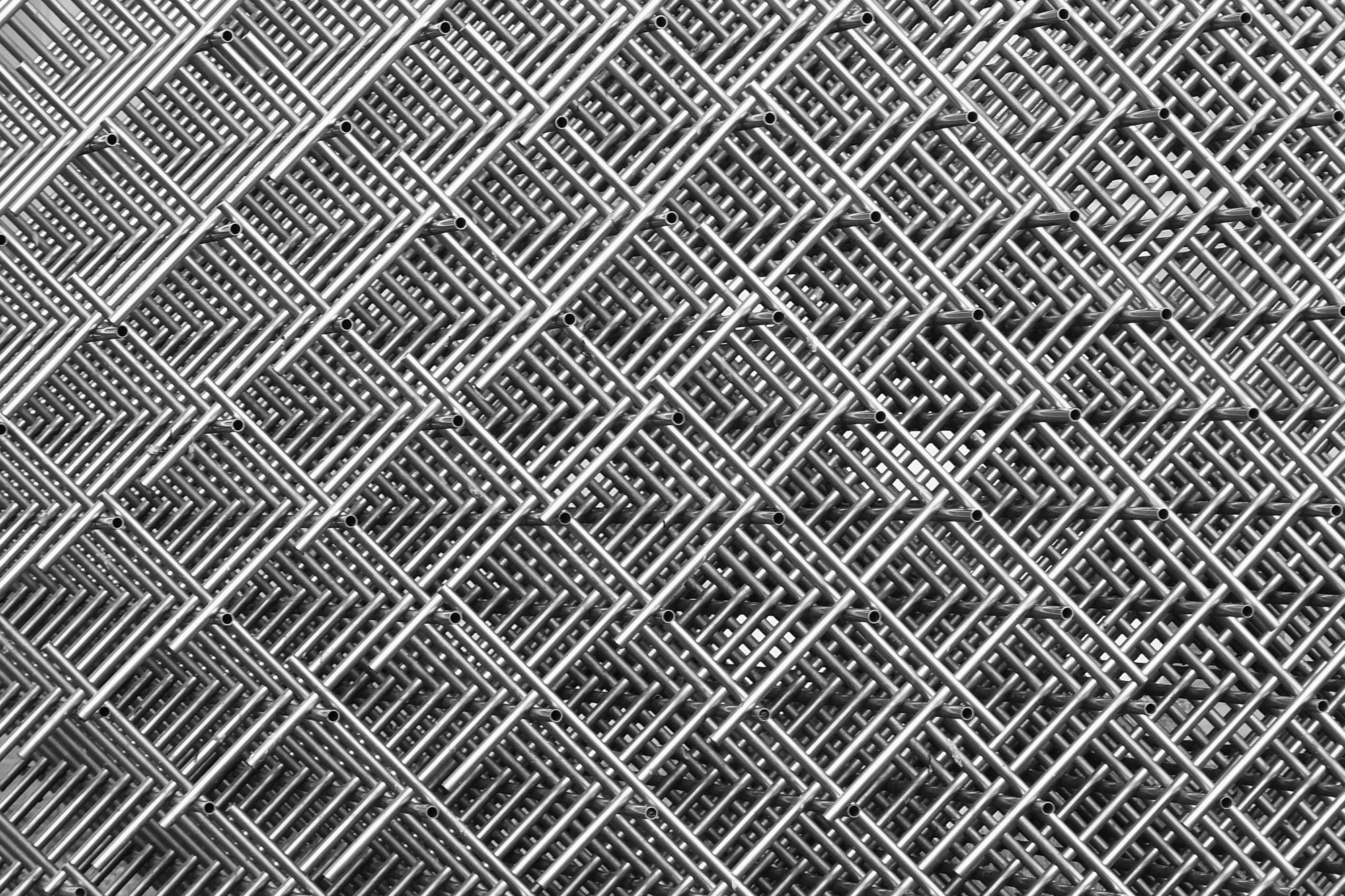
This is how scientific knowledge extends its knowledge of complex systems. Once upon a time, your humble blogger was a hydrologist studying moisture in the earth’s topsoil. Measurements are relatively simple - dig a few holes, install a few sensors, record some data, take a shower, and dig a few more holes the next day. Easy, right? Well, there’s a problem. Sensors are expensive, and flights to test sites in the US of A were expensive enough2, but what if the desired location of measurement is in Mongolia? Also, the surface of the earth is covered with a complex set of soil textures, topographies, vegetation, and climates. It would require billions of sensors to measure it all in any detail. So what’s a scientist to do? The short answer is to build models that extend the reach of sensors. If we can measure at 5cm depth, what can a model infer about 10cm and 20cm? If we can measure here, how might we estimate activity 10m over. How about 100m? What about a location 1,000km away that happens to be quite similar in terms of texture, topography, and climate? The NLB competition challenges neuroscientists to engage in a similar pursuit to extend our ability to measure the only landscape more complex than the globe - the brain.
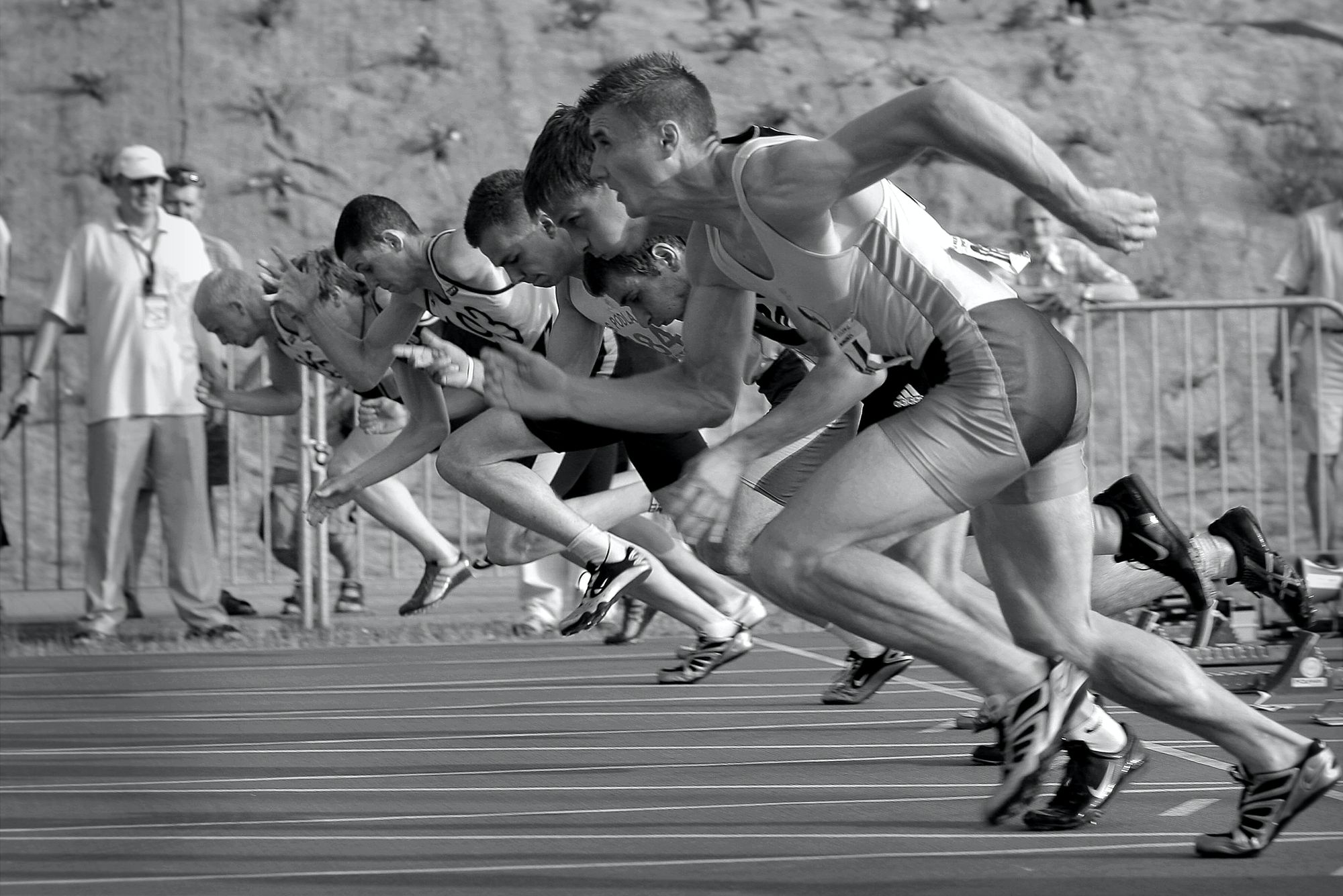
There are numerous datasets of this type, such as MC Maze, which was gathered by monitoring the neurological activity of a monkey brain solving a maze. Results from five benchmark models were provided by the competition’s administrators-in other words, take the state-of-the-art models from the most recent research papers, and throw down the gauntlet. This becomes the baseline any would-be challengers must surpass. On November 18th, 2021, the first competitor to surpass that baseline was none other than AE. Our machine learning researchers took the lead, besting the performance of the best model of neural activity known to modern science on this dataset. And if we are ultimately the best modelers when the competition concludes on January 7th, 2022, we’ll reveal the entirety of our methods. No peer-reviewed publication hidden behind a paywall, no obfuscation. Just the best approach, available to all.
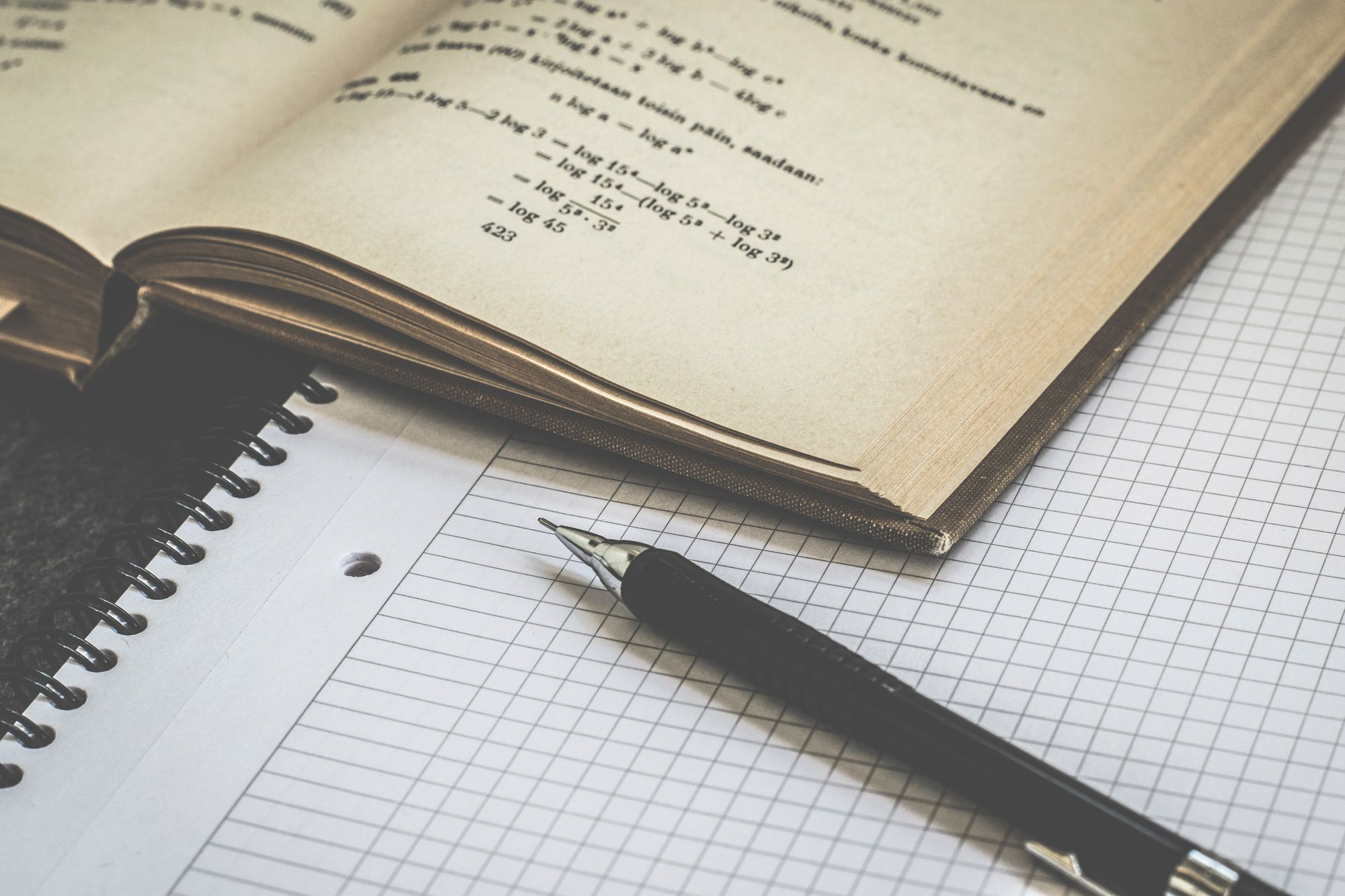
What does this mean? It means, among all machine learning outfits interested in BCI, our models are the most adept at recognizing patterns in neural data. It means we grasp the state of the art and possess the creativity and technical chops to improve upon it.
More to come, more to learn, and like any compelling competition, there will be updates and reporting. Perhaps no one is following their fantasy machine learning team on Sunday afternoons as winter comes. But give or take a few expletives lobbed at screens, there might be no competition more capable of impacting the future of our species.
Ultimately, amidst the impossible complexity of 80 billion neurons, patterns emerge. It is the ability to recognize those patterns that unlocks the potential to alleviate suffering and extend the capabilities of human beings. The tools with which those patterns are recognized are those found in the insights and mathematics of machine learning. The collaboration of AE's data scientists and neuroscience experts tops a leaderboard today, and might just increase agency for all human beings tomorrow.
AE thanks Joel Ye and Chethan Pandarinath for their work on Neural Data Transformers. Their open-source, easy-to-use codebase was foundational for the work described above (in our repo).
1
The accuracy and speed with which ideas are translated into intention and action. I have thoughts in my head, but before you can grasp those thoughts, first I must find the appropriate words, then use my inefficient fingers to type those words, then you must read those pixels upon the screen, process their meaning, and ultimately, grasp the idea I intended to convey (hopefully). The quantity of time and energy devoted to transcription fluency between human beings or between human beings and machines is staggering. What if, on a not-so-distant day, the thought, the intent, could be transcribed fluently, instantaneously, to the recipient?
2
One test site was located in Marena, OK. That municipality contained one home, which may or may not have been inhabited. Around the turn of the 20th century, the US government offered these parcels, at no cost, to any family willing to work the land and grow food. Many tried. They discovered that, even at a price of “free,” it still wasn’t worth it. They left. The government retained the land as a hydrological test site. Circa 2014, I wandered these plots on hot summer days, equipment in hand, gathering data. The science was fascinating. Oklahoma is about as dull and dusty as Steinbeck would have you believe.
No one works with an agency just because they have a clever blog. To work with my colleagues, who spend their days developing software that turns your MVP into an IPO, rather than writing blog posts, click here (Then you can spend your time reading our content from your yacht / pied-a-terre). If you can’t afford to build an app, you can always learn how to succeed in tech by reading other essays.
Building the best model of brain activity: We're Leading The Neural Latents Benchmark Challenge
"We don't quit at halftime" (But we are in the lead)
From returning agency to a human being with paralyzed limbs, to restoring auditory function, to treating depression, the concept of a brain-computer-interface (BCI) presents a mind-boggling set of possibilities. One day, rather than pounding keyboards and sliding mice to convey our intentions to our laptops, our thoughts will simply elicit the desired responses. Perfect transcription fluency1 would revolutionize how human beings invest their professional and leisure hours and redefine the term “able-bodied.”
Realizing the promise of BCI means understanding the activity of the 80 billion neurons firing in our brains, then translating the complexity of that activity into “latent” patterns. For instance, while we speak to one another, you have no idea whatsoever about the specific neurons firing in my brain, but you can discern a “latent” pattern, like the nodding of my head in agreement when you describe how a Bruce Springsteen concert is a religious experience or the visceral, snarling anger on my face when you tell me you root for the Dallas Cowboys.
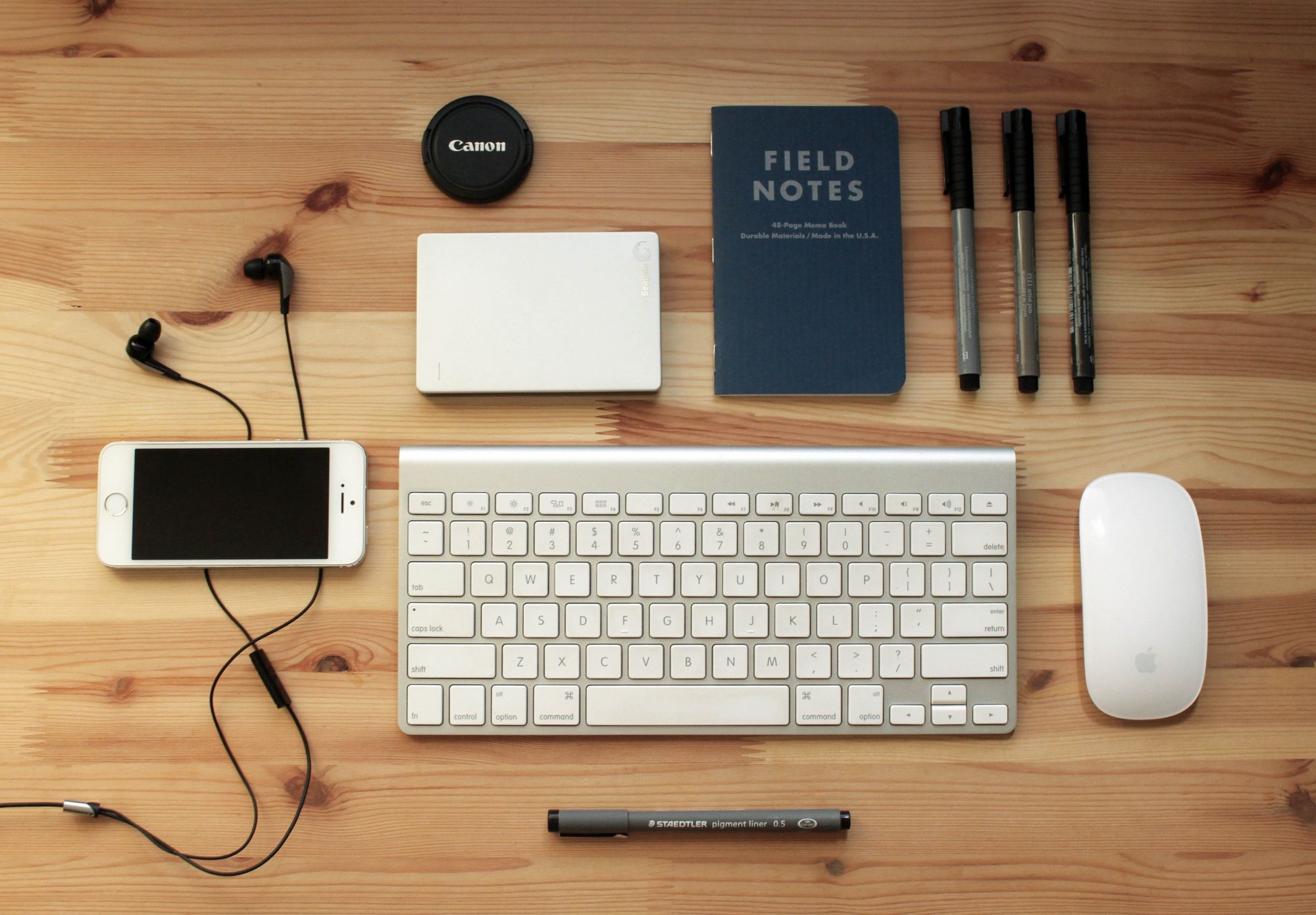
On September 5th, 2021, while most of us were fixated on the beginning of the upcoming football season, a less publicized, but perhaps more important competition began. The Neural Latents Benchmark Challenge begins with data from (primarily) Utah Arrays implanted in monkey brains. Electrodes read the behavior of 100+ monkey neurons. The mathematical challenge is simply stated: determine if an additional few dozen neurons are active or not. As an analogy, consider an enormous checkerboard grid. Now remove the colors. The grid contains 80 billion squares. You are told the color (red or black) of ~100 squares. You are instructed to determine the color (red or black) of an additional few dozen squares.
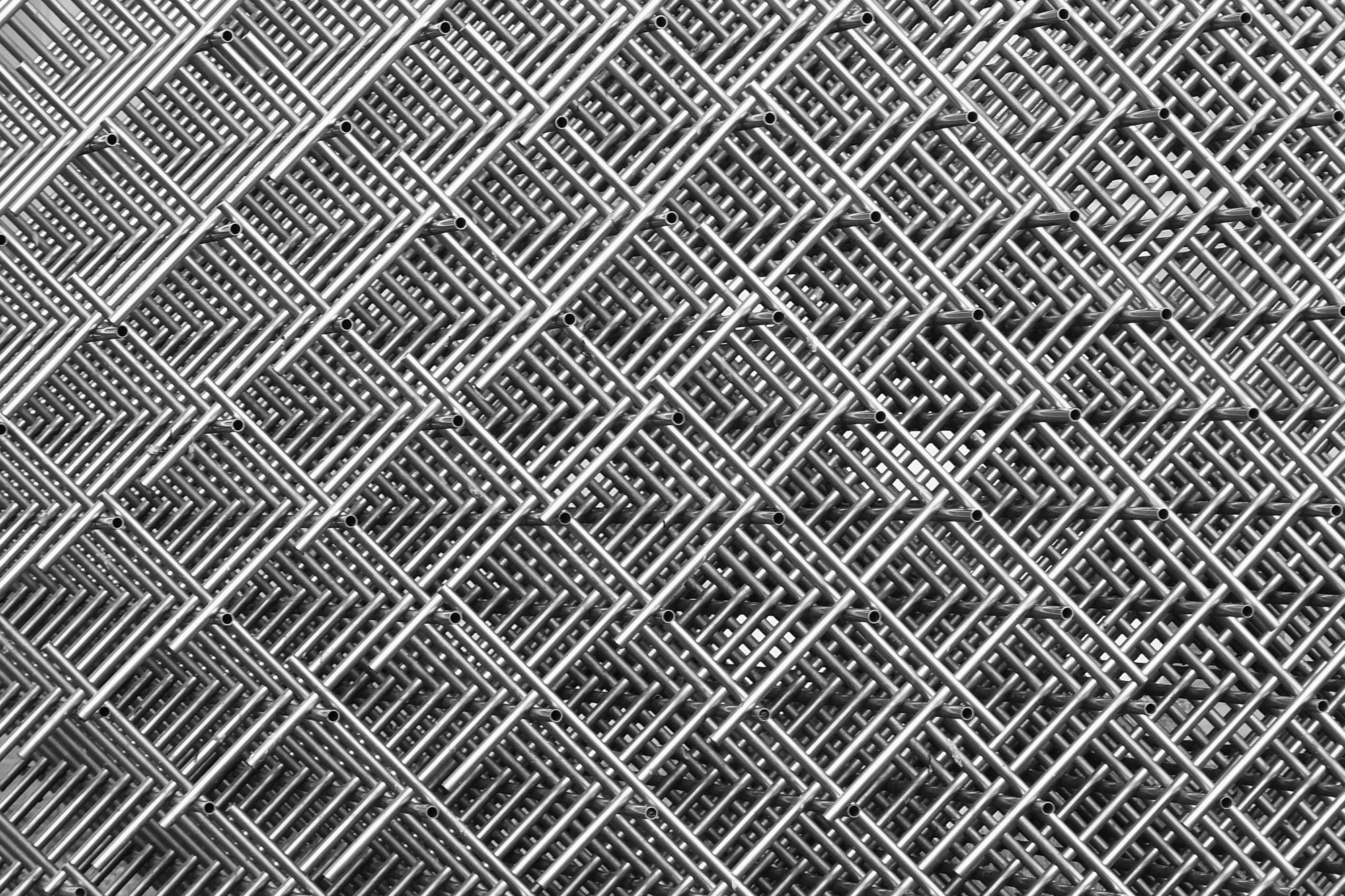
This is how scientific knowledge extends its knowledge of complex systems. Once upon a time, your humble blogger was a hydrologist studying moisture in the earth’s topsoil. Measurements are relatively simple - dig a few holes, install a few sensors, record some data, take a shower, and dig a few more holes the next day. Easy, right? Well, there’s a problem. Sensors are expensive, and flights to test sites in the US of A were expensive enough2, but what if the desired location of measurement is in Mongolia? Also, the surface of the earth is covered with a complex set of soil textures, topographies, vegetation, and climates. It would require billions of sensors to measure it all in any detail. So what’s a scientist to do? The short answer is to build models that extend the reach of sensors. If we can measure at 5cm depth, what can a model infer about 10cm and 20cm? If we can measure here, how might we estimate activity 10m over. How about 100m? What about a location 1,000km away that happens to be quite similar in terms of texture, topography, and climate? The NLB competition challenges neuroscientists to engage in a similar pursuit to extend our ability to measure the only landscape more complex than the globe - the brain.
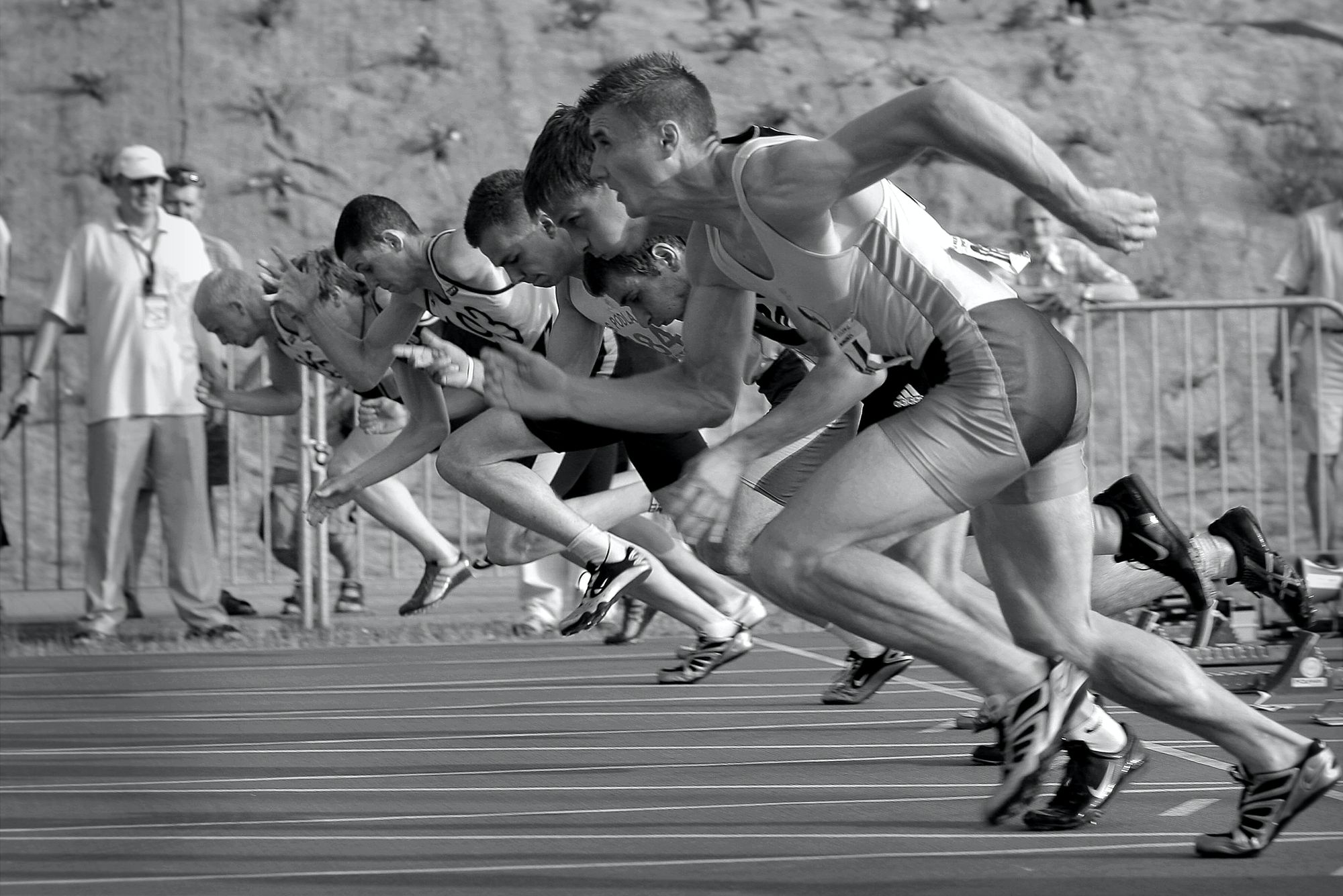
There are numerous datasets of this type, such as MC Maze, which was gathered by monitoring the neurological activity of a monkey brain solving a maze. Results from five benchmark models were provided by the competition’s administrators-in other words, take the state-of-the-art models from the most recent research papers, and throw down the gauntlet. This becomes the baseline any would-be challengers must surpass. On November 18th, 2021, the first competitor to surpass that baseline was none other than AE. Our machine learning researchers took the lead, besting the performance of the best model of neural activity known to modern science on this dataset. And if we are ultimately the best modelers when the competition concludes on January 7th, 2022, we’ll reveal the entirety of our methods. No peer-reviewed publication hidden behind a paywall, no obfuscation. Just the best approach, available to all.
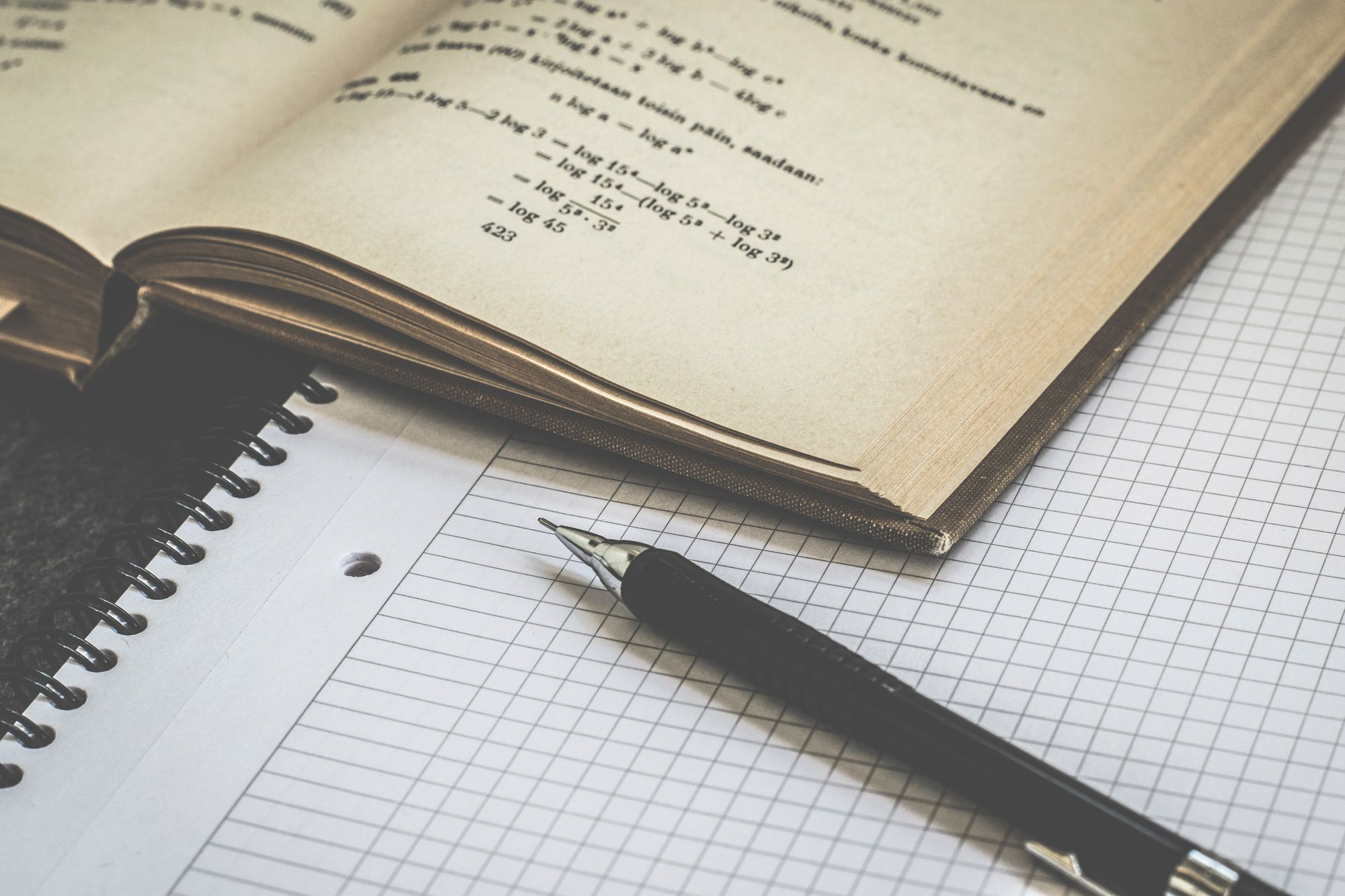
What does this mean? It means, among all machine learning outfits interested in BCI, our models are the most adept at recognizing patterns in neural data. It means we grasp the state of the art and possess the creativity and technical chops to improve upon it.
More to come, more to learn, and like any compelling competition, there will be updates and reporting. Perhaps no one is following their fantasy machine learning team on Sunday afternoons as winter comes. But give or take a few expletives lobbed at screens, there might be no competition more capable of impacting the future of our species.
Ultimately, amidst the impossible complexity of 80 billion neurons, patterns emerge. It is the ability to recognize those patterns that unlocks the potential to alleviate suffering and extend the capabilities of human beings. The tools with which those patterns are recognized are those found in the insights and mathematics of machine learning. The collaboration of AE's data scientists and neuroscience experts tops a leaderboard today, and might just increase agency for all human beings tomorrow.
AE thanks Joel Ye and Chethan Pandarinath for their work on Neural Data Transformers. Their open-source, easy-to-use codebase was foundational for the work described above (in our repo).
1
The accuracy and speed with which ideas are translated into intention and action. I have thoughts in my head, but before you can grasp those thoughts, first I must find the appropriate words, then use my inefficient fingers to type those words, then you must read those pixels upon the screen, process their meaning, and ultimately, grasp the idea I intended to convey (hopefully). The quantity of time and energy devoted to transcription fluency between human beings or between human beings and machines is staggering. What if, on a not-so-distant day, the thought, the intent, could be transcribed fluently, instantaneously, to the recipient?
2
One test site was located in Marena, OK. That municipality contained one home, which may or may not have been inhabited. Around the turn of the 20th century, the US government offered these parcels, at no cost, to any family willing to work the land and grow food. Many tried. They discovered that, even at a price of “free,” it still wasn’t worth it. They left. The government retained the land as a hydrological test site. Circa 2014, I wandered these plots on hot summer days, equipment in hand, gathering data. The science was fascinating. Oklahoma is about as dull and dusty as Steinbeck would have you believe.