The 'Neglected Approaches' Approach: AE Studio's Alignment Agenda
by Cameron Berg, Marc Carauleanu, and Judd Rosenblatt
Many thanks to Samuel Hammond, Cate Hall, Beren Millidge, Sumner Norman, Steve Byrnes, Lucius Bushnaq, Joar Skalse, Kyle Gracey, Gunnar Zarncke, Ross Nordby, David Lambert, Simeon Campos, Bogdan Ionut-Cirstea, Ryan Kidd, and Eric Ho for critical comments and suggestions on earlier drafts of this agenda, as well as Philip Gubbins, Diogo de Lucena, Rob Luke, and Mason Seale from AE Studio for their support and feedback throughout.
TL;DR
- Our initial theory of change at AE Studio was a 'neglected approach' that involved rerouting profits from our consulting business towards the development of brain-computer interface (BCI) technology to dramatically enhance human agency, better enabling us to do things like solve alignment. Now, given shortening timelines, we're updating our theory of change to scale up our technical alignment efforts.
- With a solid technical foundation in BCI, neuroscience, and machine learning, we are optimistic that we’ll be able to contribute meaningfully to AI safety. We are particularly keen on pursuing neglected technical alignment agendas that seem most creative, promising, and plausible. We are currently onboarding promising researchers and kickstarting our internal alignment team.
- As we forge ahead, we're actively soliciting expert insights from the broader alignment community and are in search of data scientists and alignment researchers who resonate with our vision of enhancing human agency and helping to solve alignment.
About us
Hi! We are AE Studio, a bootstrapped software and data science consulting business. Our mission has always been to reroute our profits directly into building technologies that have the promise of dramatically enhancing human agency, like Brain-Computer Interfaces (BCI). We also donate 5% of our revenue directly to effective charities. Today, we are ~150 programmers, product designers, and ML engineers; we are profitable and growing. We also have a team of top neuroscientists and data scientists with significant experience in developing ML solutions for leading BCI companies, and we are now leveraging our technical experience and learnings in these domains to assemble an alignment team dedicated to exploring neglected alignment research directions that draw on our expertise in BCI, data science, and machine learning.
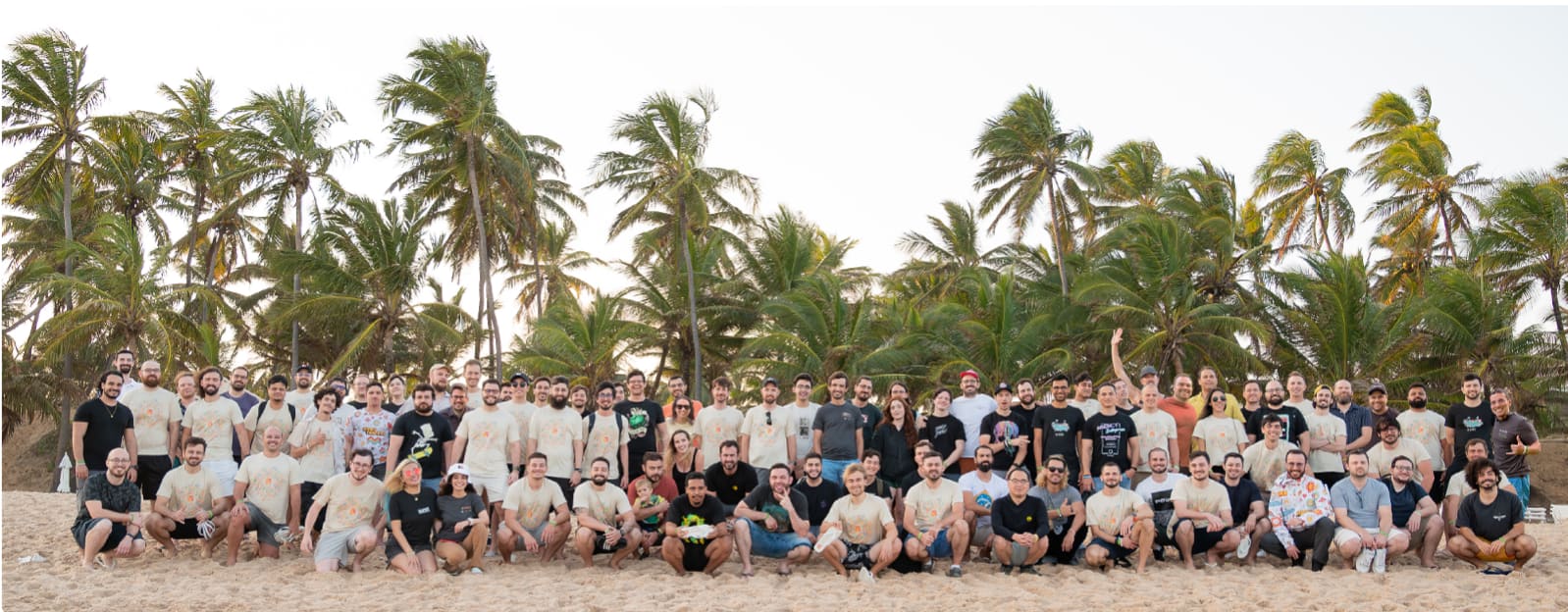
As we are becoming more public with our AI Alignment efforts, we thought it would be helpful to share our strategy and vision for how we at AE prioritize what problems to work on and how to make the best use of our comparative advantage.
Why and how we think we can help solve alignment
We can probably do with alignment what we already did with BCI
You might think that AE has no business getting involved in alignment—and we agree.
AE’s initial theory of change sought to realize a highly “neglected approach” to doing good in the world: bootstrap a profitable software consultancy, incubate our own startups on the side, sell them, and reinvest the profits in Brain Computer Interfaces (BCI) in order to do things like dramatically increase human agency, mitigate BCI-related s-risks, and make humans sufficiently intelligent, wise, and capable to do things like solve alignment. While the vision of BCI-mediated cognitive enhancement to do good in the world is increasingly common today, it was viewed as highly idiosyncratic when we first set out in 2016.
Initially, many said that AE had no business getting involved in the BCI space (and we also agreed at the time)—but after hiring leading experts in the field and taking increasingly ambitious A/B-tested steps in the right direction, we emerged as a respected player in the space (see here, here, here, and here for some examples).
Now, given accelerating AI timelines and the clear existential risks that this technology poses, we’ve now decided to leverage our technical expertise and learnings in BCI, data science, and machine learning to help solve alignment. Using the same strategic insights, technical know-how, and operational skillset that have served us well in scaling our software consultancy and BCI work—including practicing epistemic humility by soliciting substantial feedback from current experts in the field (please share yours!)—we're eager to begin exploring a diverse set of neglected alignment approaches. Some of the specific object-level alignment ideas that have emerged directly from our BCI work are detailed in the next section.
We’ve learned firsthand that the most promising projects often have low probabilities of success but extremely high potential upside, and we're now applying this core lesson to our AI alignment efforts.
We think that we can apply a similar model to alignment as we did for BCI: begin humbly,[1] and update incrementally toward excellent, expert-guided outputs.
Many shots on goal with neglected approaches
We think that the space of plausible directions for research that contributes to solving alignment is vast and that the still-probably-preparadigmatic state of alignment research means that only a small subset of this space has been satisfactorily explored. If there is a nonzero probability that currently-dominant alignment research agendas have hit upon one or many local maxima in the space of possible approaches, then we suspect that pursuing a diversified set (and/or a hodge-podge) of promising neglected approaches would afford greater exploratory coverage of this space.[2] Therefore, we are planning to adopt an optimistic and exploratory approach in pursuit of creative, plausible, and neglected alignment directions—particularly in areas where we possess a comparative advantage, like BCI and human neuroscience. We suspect many in the EA community already agree that groundbreaking innovations are often found in some highly unexpected places, seeming to many as implausible, heretical, or otherwise far-fetched—until they work.
…but what are these neglected approaches?
Your neglected approach ideas
We think we have some potentially promising hypotheses. But because we know you do, too, we are actively soliciting input from the alignment community. We will be more formally pursuing this initiative in the near future, awarding some small prizes to the most promising expert-reviewed suggestions. Please submit any[3] agenda idea that you think is both plausible and neglected (even if you don’t have the bandwidth right now to pursue the idea! This is a contest for ideas, not for implementation).
Our neglected approach ideas
To be clear about our big-picture goal: we want to ensure that if/when we live in a world with superintelligent AI whose behavior is—likely by definition—outside our direct control, this AI (at the very least) does not destroy humanity and (ideally) dramatically increases the agency and flourishing of conscious entities.
Accordingly, the following list presents a set of ten ideas that we think (1) have some reasonable probability of contributing to the realization of this vision, (2) have not been explored satisfactorily, and (3) we could meaningfully contribute to actualizing.
Important caveats
- Please consider this set of ideas something far more like ‘AE’s evolving, first-pass best guesses at promising neglected alignment approaches’ rather than ‘AE’s official alignment agenda.’
- Please also note that these are our ideas, not concrete implementation plans. While we think we might have a comparative advantage in pursuing some of the following agendas, we do not think this is likely to be the case across the board; we see the following ideas as generally-interesting, definitely-neglected, alignment-related agendas—even if we aren’t the specific group that is best suited to implement all of them.
- One meta-approach we are exploring involves quantitatively identifying neglected approaches, such as analyzing a very large natural language dataset of alignment research. We suspect this and other related projects may be instrumental in identifying specific research areas that are currently underrepresented.
Ten examples of neglected approaches we think are probably worth pursuing
- Reverse-engineering prosociality: We agree that humans provide an untapped wealth of evidence about alignment. The neural networks of the human brain robustly instantiate prosocial algorithms such as empathy, self-other overlap, theory of mind, attention schema, self-awareness, self-criticism, self-control, humility, altruism and more. We want to reverse-engineer—and contribute to further developing—our current best models of how prosociality happens in the brain, toward the construction of robustly prosocial AI. With AE's background in BCI, neuroscience, and machine learning, we feel well-equipped to make tangible progress in this research direction.
- We are currently actively working on operationalizing attention schema theory, self-other overlap, and theory of mind for RL- and LLM-based agents as mechanisms for facilitating prosocial cognition. Brain-based approaches to AI have proven to be generally successful for AI capabilities research, and we (along with many others) think the same is likely to be true for AI safety. We are interested in testing the hypothesis that prosocial learning algorithms are more performant and scalable as compared to default approaches. We also think that creating and/or facilitating the development of relevant benchmarks and datasets might be a very high leverage subproject associated with this approach.
- Though we are aware that current models of human prosociality are far from perfect, we believe that the associated scientific literature is a largely untapped source of inspiration both for (1) what sort of incentives and mechanisms make agents prosocial, and (2) under what conditions prosociality robustly contributes to aligned behavior. We think this existing work is likely to inspire novel alignment approaches in spite of the certainly-still-imperfect nature of computational cognitive neuroscience.
- Best guesses for why this might be neglected:
- We speculate that there may be a tendency to conflate (1) the extraction of the best alignment-relevant insights from cognitive neuroscience (we support this), with (2) the assumption that AGI will mimic the human brain (we don’t think this is likely), or (3) the idea that we already have perfect models from current neuroscience of how prosociality works (this is empirically not true), or (4) that we should in all cases try to replicate the social behavior of human brains in AI (we think this is unwise and unsafe)—all of which has needlessly limited the extent to which (1) has been pursued.
- Additionally, the alignment community's strong foundation in mathematics, computer science, and other key technical fields, while undeniably valuable, may inadvertently limit community-level exposure to the cutting edge of cognitive science research.
- Transformative AI → better BCI → better (human) alignment researchers: Some alignment researchers want to employ advanced AI to automate and/or rapidly advance alignment research directly (most prominently, OpenAI’s Superalignment agenda). We think there is a highly neglected direction to pursue in this same vein: employ advanced AI to automate and/or rapidly advance BCI research. Then, use this BCI to dramatically augment the capabilities of human alignment researchers.
- While this may sound somewhat outlandish, we suspect that significant scientific automation is plausible in the near future, and we want to flag that there are other potentially-very-high-value alignment directions that emerge from this breakthrough besides directly jumping to automating alignment research—including automating things like connectomics/whole brain emulation. (Incidentally, we also think it's worth considering various other benefits of transformative AI for a safer post-AGI future, such as effectively encrypting human DNA with unique DNA codons to combat biorisk.)
- It is also worth noting that augmenting the capabilities of human alignment researchers does not necessarily require transformative BCI; to this end, we are currently investigating relatively-lower-hanging psychological interventions and agency-enhancing tools that have the potential to significantly enhance the quality and quantity of individuals’ cognitive output. In an ideal world (i.e., one where we can begin implementing this agenda reasonably quickly), we speculate it might be safer to empower humans to do better alignment research than AI, as empowering AI carries alignment-relevant capabilities risks that empowering humans does not (which also is not to say that empowering humans via BCI does also not have many serious risks).
- BCI for quantitatively mapping human values: we also think that near-future-BCI may enable us to map the latent space of human values in a far more data-driven way than, for instance, encoding our values in natural language, as is the case, for instance, in Anthropic’s constitutional AI. This research is already happening in a more constrained way—we suspect that BCI explicitly tailored to mapping cognition related to valuation would be very valuable for alignment (to individuals, groups, societies, etc.).
- ‘Reinforcement Learning from Neural Feedback’ (RLNF): near-future BCI may also allow us to interface neural feedback directly with AI systems, enabling us to improve the alignment of the state-of-the-art reward prediction models (and/or develop novel reward models altogether) in the direction of yielding more efficient, individually-tailored, high-fidelity reward signals. We think that in order for this approach to be pragmatic, the increase in quality of the reward signals would have to outweigh or otherwise counterbalance the practical cost of extracting the associated brain signals.[4] And the general idea of using neural data as an ML training signal also need not be limited to RL—we just thought RLNF sounded pretty cool.
- Provably safe architectures:[5] we see significant potential to help amplify, expedite, and scale the deployment of provably safe architectures, including potentially promising examples like open agency architectures, inductive program synthesis (e.g., DreamCoder), and other similar frameworks that draw on insights from cognitive neuroscience. Though these architectures are not currently prominent in machine learning, we think it is possible that devoting effort and resources to scaling them up for mainstream adoption could potentially be highly beneficial in expectation. We are sensitive to the concern that the alignment tax might be high in adopting uncompetitive architectures—which is precisely why we think these architectures deserve more rather than less technical attention and funding.
- Intelligent field-building as an indirect alignment approach:[6] despite the increasing mainstream ubiquity of AI safety research, there is still only a tiny subset of smart and experienced people who could very likely add value to alignment who are in fact currently doing so. If we can carefully identify these extremely promising thinkers—especially those from disciplines and backgrounds that may be traditionally overlooked (e.g., neuroscience)—and get them into a state where they can contribute meaningfully to alignment, we think that this could enable us to develop, test, and iterate on unconventional approaches at scale.
- Facilitate the development of explicitly-safety-focused businesses: as alignment efforts become increasingly mainstream, we suspect that AI safety frameworks may yield innovations upon which various promising business models may be built. We also think it would be a far better outcome if, all else being equal, more emerging for-profit AI companies decide to build alignment-related products (rather than build products that just further advance capabilities, which seems like the current default behavior). We suspect many capable startup founders could be nerd sniped into doing something more impactful with alignment.
- Some plausible examples of such businesses could include (1) consultancies offering red-teaming as a service for adversarial testing of AI systems, (2) platforms providing robust testing/benchmarking/auditing software for advanced AI systems, (3) centralized services that deliver high-quality, expert-labeled, ethically-sourced datasets for unbiased ML training, and (4) AI monitoring services akin to Datadog for continuous safety and performance tracking. We know of several founders currently setting out to pursue similarly safety-focused business models.
- Accordingly, we are planning to do all the following:
- we’re currently growing a community of VCs and angels interested in funding such ideas,
- starting Q2 next year, we aim to fund, internally develop, and deploy safety-prioritizing AI skunkworks companies (ideally as an exportable model for others to follow), and
- we're planning to run a competition with $50K seed funding for already-existing safety-focused businesses and/or anyone who has promising business ideas that first and foremost advance alignment, to be evaluated by AI safety experts and concerned business leaders. We do encourage you to post any promising ideas, even if you're not likely to pursue them.
- We also suspect it may be worth creating some template best practices with company formation to increase the likelihood that the businesses retain agency long term in accomplishing AI safety goals, especially given recent events. Aligning business interests with public safety is not just beneficial for societal welfare, but also advantageous for long-term business sustainability—as well as potentially influencing public perception and policy efforts in a dramatically positive way. We also are acutely aware of safety-washing concerns and/or unintentionally creating race dynamics in this domain, and we think that ensuring for-profit safety work is technically rigorous and productive is critical to get right.
- If you are a potential funder for promising businesses that advance alignment, please reach out to us at alignmentangels@ae.studio to express interest in joining our Alignment Angels slack group.
- Scaling our consulting business to do object-level technical alignment work—and then scale this model to many other organizations: the potential to bring other highly promising people into the fold (see point 6, above) to contribute significantly to alignment—even without being alignment experts per se—is a hypothesis we're actively exploring and aiming to validate.
- Given that we expect most people to struggle with having actually-impactful alignment outputs as they are just starting, we see a model where senior AI engineers—even those without explicit alignment backgrounds—can eventually collaborate with a small number of extremely promising alignment researchers who have an abundance of excellent object-level technical project ideas but limited capacity to pursue them. By integrating these researchers into our client engagement framework, used highly successfully over the years for our other technical projects, we could potentially massively scale the efficacy of these researchers, leveraging our team's extensive technical expertise to advance these alignment projects and drive meaningful progress in the field.
- We hope that if this ‘outsource-specific-promising-technical-alignment-projects’ model works, many other teams (corporations, nonprofits, etc.) with technical talent would copy it—especially if grants are made in the future to further enable this approach.
- Neuroscience x mechanistic interpretability: both domains have yielded insights that are mutually elucidating for the shared project of attempting to model how neural data leads to complex cognitive properties. We think it makes a lot of sense to put leading neuroscientists in conversation with mechanistic interpretability researchers in an explicit and systematic way, such that the cutting-edge methods in each discipline can be further leveraged to enhance the other. Of course, we think that this synergy across research domains should be explicitly focused on enhancing safety and interpretability rather than using neuroscience insights to extend AI capabilities.
- Neglected approaches to AI policy—e.g., lobby government to directly fund alignment research: though not a technical research direction, we think that this perspective dovetails nicely with other thinking-outside-the-box alignment approaches that we’ve shared here. It appears as though congresspeople and staffers are taking the alignment problem more seriously than many would have initially predicted and, in particular, are quite open to plausible safety proposals—all of which means that there may be substantial opportunity to capitalize on the vast funding resources at their disposal to dramatically increase the scale and speed at which alignment work is being done. We think it is critical to make sure that this is done effectively and efficiently (e.g., avoiding pork) and for alignment organizations to be practically prepared to manage and utilize significant investment (e.g., 10-1000x) if such funding does in fact come to fruition in the near future. We are currently exploring the possibility of hiring someone with a strong policy background to help facilitate this: while we have received positive feedback on this general idea from those who know significantly more about the policy space than we do, we are very sensitive to the potential for a shortsighted or naive implementation of this to be highly harmful to AI safety policy. We are actively in the process of meeting with and learning more from policy experts: if you are doing work in this area and know way more than us about AI policy, please do reach out so we can learn from you!
We began sharing this ‘Neglected Approaches’ approach publicly at the Foresight Institute’s Whole Brain Emulation Workshop in May, and we were excited to see this strategy gain steam, including Foresight Institute’s own emphasis on neglected approaches with their new Grant for Underexplored Approaches to AI Safety.
We want to make these ideas stronger
It is critical to emphasize again that this list represents our current best guesses on some plausible neglected approaches that we think we are well-equipped to explore further. We fully acknowledge that many of these guesses may be ill-conceived for some reason we haven’t anticipated and are open to critical feedback in order to make our contributions as positively impactful as possible. We intend to keep the community updated with respect to our working models and plans for contributing maximally effectively to alignment. (Please see this feedback form if you’d prefer to share your thoughts on our work anonymously/privately instead of leaving a comment below this post.)
We also recognize that many of these proposals have a double-edged sword quality that requires extremely careful consideration—e.g., building BCI that makes humans more competent could also make bad actors more competent, give AI systems manipulation-conducive information about the processes of our cognition that we don’t even know, and so on. We take these risks very seriously and think that any well-defined alignment agenda must also put forward a convincing plan for avoiding them (with full knowledge of the fact that if they can’t be avoided, they are not viable directions.)
Concluding thoughts
AE Studio's burgeoning excitement about contributing to AI safety research is a calculated response to our updated timelines and optimism about having the skillset required for making impactful contributions. Our approach aims to combine our expertise in software, neuroscience, and data science with ambitious parallel exploration of what we consider to be neglected approaches in AI alignment.
We commit to exploring these directions in a pragmatic, informed, and data-driven manner, emphasizing collaboration and openness within the greater alignment community. We care deeply about contributing to alignment because we want to bring about a maximally agency-increasing future for humanity—and without the precondition of robustly aligned AGI, this future seems otherwise impossible to attain.
We are actively hiring for senior alignment researchers to join/advise our team, in addition to data scientists with a neuroscience background, those highly experienced in policymaker engagement, and a number of other positions. If you have any other questions, comments, or ideas, please do reach out.
[1] Miscellaneous cool accomplishment: before we started getting involved in AI safety in any serious way, two AE engineers with no prior background in alignment developed a framework for studying prompt injection attacks that went on to win Best Paper at the 2022 NeurIPS ML Safety Workshop.
[2] To illustrate this point more precisely, we can consider a highly simplified probabilistic model of the research space. (We recognize this sort of neglect math is likely highly familiar to many EAs, and we don't mean to be pedantic by including it; we've put it here because we think it is a succinct way of demonstrating—if only to ourselves—why taking on multiple neglected approaches is rational.)
Let’s say the total number of plausible alignment agendas is \( n \). Let’s stipulate that currently, alignment researchers have meaningfully explored \( k \) approaches, meaning that \( n-k \) approaches remain unexplored. (As stated previously, we suspect that current mainstream alignment research is likely exploiting only a small subset of the total space of plausible alignment approaches, rendering a large number of alignment strategies either completely or mostly unexplored—i.e., we think that \( n-k \) is large.) Each neglected approach, \( i \), has a very small but nonzero probability \( p_{\text{neglect}_i} \) of being crucial for making significant progress in alignment. Treating these probabilities as independent for the sake of simplicity, the chance that all n−k neglected approaches are not key is \( \prod_{i=1}^{n-k} (1 - p_{\text{neglect}_i}) \). Conversely, the probability that at least one neglected approach is key is \( 1 - \prod_{i=1}^{n-k} (1 - p_{\text{neglect}_i}) \). This implies—at least in our simplified model—that even with low individual probabilities, a sufficiently large number of neglected approaches can collectively hold a high chance of including a crucial solution in expectation. For instance, in a world with 100 neglected approaches and a probability of 99% that each approach is not key (i.e., a 1% likelihood of pushing the needle on alignment), there’s still about a 63% chance that one of these approaches would be crucial; with 1000 approaches and a probability of 99% that each approach is not key, the probability rises to over 99% that one will be pivotal. This simple model motivates us to think it makes sense to take many shots on goal, pursuing as many plausible neglected alignment agendas as possible.
[3] Please note: (1) we are primarily interested in aggregating the best ideas to begin, so don’t worry if you have an idea that you think fits the criteria above but is challenging to implement/you wouldn’t want to actually implement it. (2) There is space on the form to denote that your suggested approach is exfohazardous.
[4] This is a core trade-off in our work and something that we have made substantial progress on since our founding.
[5] We want to call out that this approach is likely the least neglected of the ten we enumerate here—which is not to say it isn’t neglected in an absolute sense.
[6] While there are a good number of newer organizations working on fieldbuilding for alignment, we think it remains highly neglected given the potential impact, especially in likely-impactful fields that are only now starting to be considered within the Overton window.
The 'Neglected Approaches' Approach: AE Studio's Alignment Agenda
by Cameron Berg, Marc Carauleanu, and Judd Rosenblatt
Many thanks to Samuel Hammond, Cate Hall, Beren Millidge, Sumner Norman, Steve Byrnes, Lucius Bushnaq, Joar Skalse, Kyle Gracey, Gunnar Zarncke, Ross Nordby, David Lambert, Simeon Campos, Bogdan Ionut-Cirstea, Ryan Kidd, and Eric Ho for critical comments and suggestions on earlier drafts of this agenda, as well as Philip Gubbins, Diogo de Lucena, Rob Luke, and Mason Seale from AE Studio for their support and feedback throughout.
TL;DR
- Our initial theory of change at AE Studio was a 'neglected approach' that involved rerouting profits from our consulting business towards the development of brain-computer interface (BCI) technology to dramatically enhance human agency, better enabling us to do things like solve alignment. Now, given shortening timelines, we're updating our theory of change to scale up our technical alignment efforts.
- With a solid technical foundation in BCI, neuroscience, and machine learning, we are optimistic that we’ll be able to contribute meaningfully to AI safety. We are particularly keen on pursuing neglected technical alignment agendas that seem most creative, promising, and plausible. We are currently onboarding promising researchers and kickstarting our internal alignment team.
- As we forge ahead, we're actively soliciting expert insights from the broader alignment community and are in search of data scientists and alignment researchers who resonate with our vision of enhancing human agency and helping to solve alignment.
About us
Hi! We are AE Studio, a bootstrapped software and data science consulting business. Our mission has always been to reroute our profits directly into building technologies that have the promise of dramatically enhancing human agency, like Brain-Computer Interfaces (BCI). We also donate 5% of our revenue directly to effective charities. Today, we are ~150 programmers, product designers, and ML engineers; we are profitable and growing. We also have a team of top neuroscientists and data scientists with significant experience in developing ML solutions for leading BCI companies, and we are now leveraging our technical experience and learnings in these domains to assemble an alignment team dedicated to exploring neglected alignment research directions that draw on our expertise in BCI, data science, and machine learning.
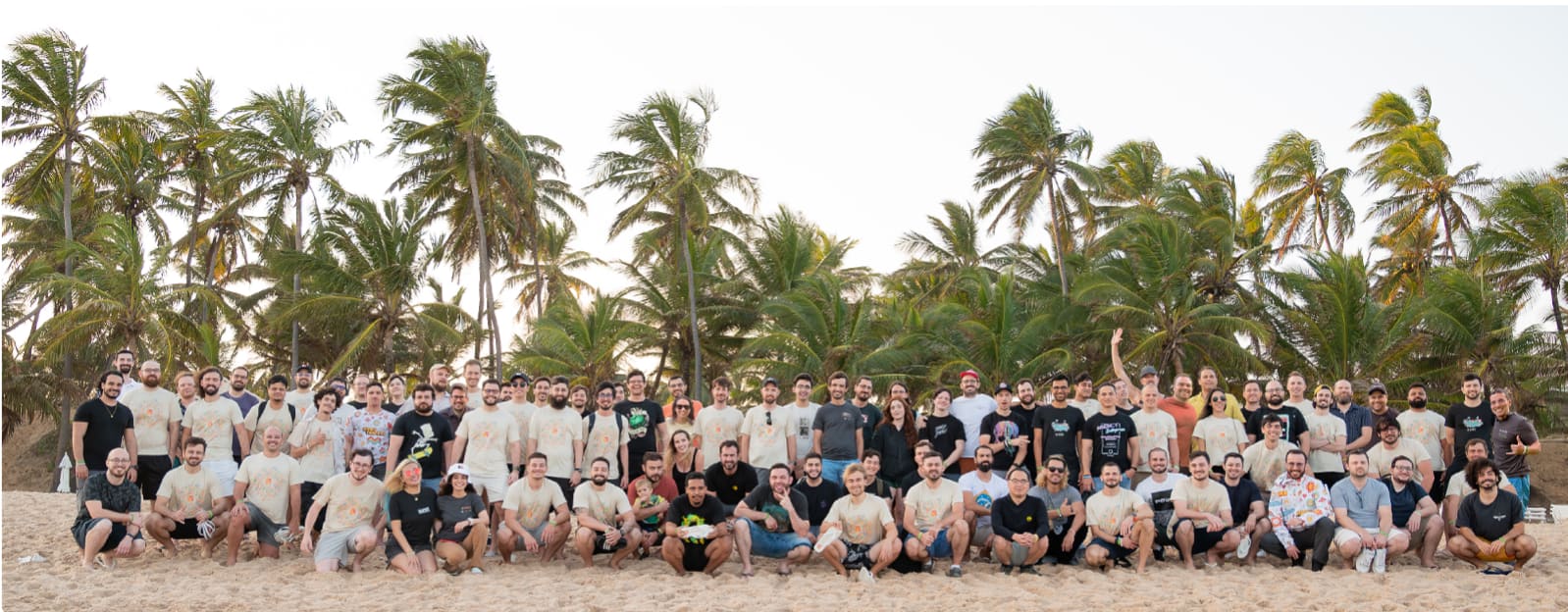
As we are becoming more public with our AI Alignment efforts, we thought it would be helpful to share our strategy and vision for how we at AE prioritize what problems to work on and how to make the best use of our comparative advantage.
Why and how we think we can help solve alignment
We can probably do with alignment what we already did with BCI
You might think that AE has no business getting involved in alignment—and we agree.
AE’s initial theory of change sought to realize a highly “neglected approach” to doing good in the world: bootstrap a profitable software consultancy, incubate our own startups on the side, sell them, and reinvest the profits in Brain Computer Interfaces (BCI) in order to do things like dramatically increase human agency, mitigate BCI-related s-risks, and make humans sufficiently intelligent, wise, and capable to do things like solve alignment. While the vision of BCI-mediated cognitive enhancement to do good in the world is increasingly common today, it was viewed as highly idiosyncratic when we first set out in 2016.
Initially, many said that AE had no business getting involved in the BCI space (and we also agreed at the time)—but after hiring leading experts in the field and taking increasingly ambitious A/B-tested steps in the right direction, we emerged as a respected player in the space (see here, here, here, and here for some examples).
Now, given accelerating AI timelines and the clear existential risks that this technology poses, we’ve now decided to leverage our technical expertise and learnings in BCI, data science, and machine learning to help solve alignment. Using the same strategic insights, technical know-how, and operational skillset that have served us well in scaling our software consultancy and BCI work—including practicing epistemic humility by soliciting substantial feedback from current experts in the field (please share yours!)—we're eager to begin exploring a diverse set of neglected alignment approaches. Some of the specific object-level alignment ideas that have emerged directly from our BCI work are detailed in the next section.
We’ve learned firsthand that the most promising projects often have low probabilities of success but extremely high potential upside, and we're now applying this core lesson to our AI alignment efforts.
We think that we can apply a similar model to alignment as we did for BCI: begin humbly,[1] and update incrementally toward excellent, expert-guided outputs.
Many shots on goal with neglected approaches
We think that the space of plausible directions for research that contributes to solving alignment is vast and that the still-probably-preparadigmatic state of alignment research means that only a small subset of this space has been satisfactorily explored. If there is a nonzero probability that currently-dominant alignment research agendas have hit upon one or many local maxima in the space of possible approaches, then we suspect that pursuing a diversified set (and/or a hodge-podge) of promising neglected approaches would afford greater exploratory coverage of this space.[2] Therefore, we are planning to adopt an optimistic and exploratory approach in pursuit of creative, plausible, and neglected alignment directions—particularly in areas where we possess a comparative advantage, like BCI and human neuroscience. We suspect many in the EA community already agree that groundbreaking innovations are often found in some highly unexpected places, seeming to many as implausible, heretical, or otherwise far-fetched—until they work.
…but what are these neglected approaches?
Your neglected approach ideas
We think we have some potentially promising hypotheses. But because we know you do, too, we are actively soliciting input from the alignment community. We will be more formally pursuing this initiative in the near future, awarding some small prizes to the most promising expert-reviewed suggestions. Please submit any[3] agenda idea that you think is both plausible and neglected (even if you don’t have the bandwidth right now to pursue the idea! This is a contest for ideas, not for implementation).
Our neglected approach ideas
To be clear about our big-picture goal: we want to ensure that if/when we live in a world with superintelligent AI whose behavior is—likely by definition—outside our direct control, this AI (at the very least) does not destroy humanity and (ideally) dramatically increases the agency and flourishing of conscious entities.
Accordingly, the following list presents a set of ten ideas that we think (1) have some reasonable probability of contributing to the realization of this vision, (2) have not been explored satisfactorily, and (3) we could meaningfully contribute to actualizing.
Important caveats
- Please consider this set of ideas something far more like ‘AE’s evolving, first-pass best guesses at promising neglected alignment approaches’ rather than ‘AE’s official alignment agenda.’
- Please also note that these are our ideas, not concrete implementation plans. While we think we might have a comparative advantage in pursuing some of the following agendas, we do not think this is likely to be the case across the board; we see the following ideas as generally-interesting, definitely-neglected, alignment-related agendas—even if we aren’t the specific group that is best suited to implement all of them.
- One meta-approach we are exploring involves quantitatively identifying neglected approaches, such as analyzing a very large natural language dataset of alignment research. We suspect this and other related projects may be instrumental in identifying specific research areas that are currently underrepresented.
Ten examples of neglected approaches we think are probably worth pursuing
- Reverse-engineering prosociality: We agree that humans provide an untapped wealth of evidence about alignment. The neural networks of the human brain robustly instantiate prosocial algorithms such as empathy, self-other overlap, theory of mind, attention schema, self-awareness, self-criticism, self-control, humility, altruism and more. We want to reverse-engineer—and contribute to further developing—our current best models of how prosociality happens in the brain, toward the construction of robustly prosocial AI. With AE's background in BCI, neuroscience, and machine learning, we feel well-equipped to make tangible progress in this research direction.
- We are currently actively working on operationalizing attention schema theory, self-other overlap, and theory of mind for RL- and LLM-based agents as mechanisms for facilitating prosocial cognition. Brain-based approaches to AI have proven to be generally successful for AI capabilities research, and we (along with many others) think the same is likely to be true for AI safety. We are interested in testing the hypothesis that prosocial learning algorithms are more performant and scalable as compared to default approaches. We also think that creating and/or facilitating the development of relevant benchmarks and datasets might be a very high leverage subproject associated with this approach.
- Though we are aware that current models of human prosociality are far from perfect, we believe that the associated scientific literature is a largely untapped source of inspiration both for (1) what sort of incentives and mechanisms make agents prosocial, and (2) under what conditions prosociality robustly contributes to aligned behavior. We think this existing work is likely to inspire novel alignment approaches in spite of the certainly-still-imperfect nature of computational cognitive neuroscience.
- Best guesses for why this might be neglected:
- We speculate that there may be a tendency to conflate (1) the extraction of the best alignment-relevant insights from cognitive neuroscience (we support this), with (2) the assumption that AGI will mimic the human brain (we don’t think this is likely), or (3) the idea that we already have perfect models from current neuroscience of how prosociality works (this is empirically not true), or (4) that we should in all cases try to replicate the social behavior of human brains in AI (we think this is unwise and unsafe)—all of which has needlessly limited the extent to which (1) has been pursued.
- Additionally, the alignment community's strong foundation in mathematics, computer science, and other key technical fields, while undeniably valuable, may inadvertently limit community-level exposure to the cutting edge of cognitive science research.
- Transformative AI → better BCI → better (human) alignment researchers: Some alignment researchers want to employ advanced AI to automate and/or rapidly advance alignment research directly (most prominently, OpenAI’s Superalignment agenda). We think there is a highly neglected direction to pursue in this same vein: employ advanced AI to automate and/or rapidly advance BCI research. Then, use this BCI to dramatically augment the capabilities of human alignment researchers.
- While this may sound somewhat outlandish, we suspect that significant scientific automation is plausible in the near future, and we want to flag that there are other potentially-very-high-value alignment directions that emerge from this breakthrough besides directly jumping to automating alignment research—including automating things like connectomics/whole brain emulation. (Incidentally, we also think it's worth considering various other benefits of transformative AI for a safer post-AGI future, such as effectively encrypting human DNA with unique DNA codons to combat biorisk.)
- It is also worth noting that augmenting the capabilities of human alignment researchers does not necessarily require transformative BCI; to this end, we are currently investigating relatively-lower-hanging psychological interventions and agency-enhancing tools that have the potential to significantly enhance the quality and quantity of individuals’ cognitive output. In an ideal world (i.e., one where we can begin implementing this agenda reasonably quickly), we speculate it might be safer to empower humans to do better alignment research than AI, as empowering AI carries alignment-relevant capabilities risks that empowering humans does not (which also is not to say that empowering humans via BCI does also not have many serious risks).
- BCI for quantitatively mapping human values: we also think that near-future-BCI may enable us to map the latent space of human values in a far more data-driven way than, for instance, encoding our values in natural language, as is the case, for instance, in Anthropic’s constitutional AI. This research is already happening in a more constrained way—we suspect that BCI explicitly tailored to mapping cognition related to valuation would be very valuable for alignment (to individuals, groups, societies, etc.).
- ‘Reinforcement Learning from Neural Feedback’ (RLNF): near-future BCI may also allow us to interface neural feedback directly with AI systems, enabling us to improve the alignment of the state-of-the-art reward prediction models (and/or develop novel reward models altogether) in the direction of yielding more efficient, individually-tailored, high-fidelity reward signals. We think that in order for this approach to be pragmatic, the increase in quality of the reward signals would have to outweigh or otherwise counterbalance the practical cost of extracting the associated brain signals.[4] And the general idea of using neural data as an ML training signal also need not be limited to RL—we just thought RLNF sounded pretty cool.
- Provably safe architectures:[5] we see significant potential to help amplify, expedite, and scale the deployment of provably safe architectures, including potentially promising examples like open agency architectures, inductive program synthesis (e.g., DreamCoder), and other similar frameworks that draw on insights from cognitive neuroscience. Though these architectures are not currently prominent in machine learning, we think it is possible that devoting effort and resources to scaling them up for mainstream adoption could potentially be highly beneficial in expectation. We are sensitive to the concern that the alignment tax might be high in adopting uncompetitive architectures—which is precisely why we think these architectures deserve more rather than less technical attention and funding.
- Intelligent field-building as an indirect alignment approach:[6] despite the increasing mainstream ubiquity of AI safety research, there is still only a tiny subset of smart and experienced people who could very likely add value to alignment who are in fact currently doing so. If we can carefully identify these extremely promising thinkers—especially those from disciplines and backgrounds that may be traditionally overlooked (e.g., neuroscience)—and get them into a state where they can contribute meaningfully to alignment, we think that this could enable us to develop, test, and iterate on unconventional approaches at scale.
- Facilitate the development of explicitly-safety-focused businesses: as alignment efforts become increasingly mainstream, we suspect that AI safety frameworks may yield innovations upon which various promising business models may be built. We also think it would be a far better outcome if, all else being equal, more emerging for-profit AI companies decide to build alignment-related products (rather than build products that just further advance capabilities, which seems like the current default behavior). We suspect many capable startup founders could be nerd sniped into doing something more impactful with alignment.
- Some plausible examples of such businesses could include (1) consultancies offering red-teaming as a service for adversarial testing of AI systems, (2) platforms providing robust testing/benchmarking/auditing software for advanced AI systems, (3) centralized services that deliver high-quality, expert-labeled, ethically-sourced datasets for unbiased ML training, and (4) AI monitoring services akin to Datadog for continuous safety and performance tracking. We know of several founders currently setting out to pursue similarly safety-focused business models.
- Accordingly, we are planning to do all the following:
- we’re currently growing a community of VCs and angels interested in funding such ideas,
- starting Q2 next year, we aim to fund, internally develop, and deploy safety-prioritizing AI skunkworks companies (ideally as an exportable model for others to follow), and
- we're planning to run a competition with $50K seed funding for already-existing safety-focused businesses and/or anyone who has promising business ideas that first and foremost advance alignment, to be evaluated by AI safety experts and concerned business leaders. We do encourage you to post any promising ideas, even if you're not likely to pursue them.
- We also suspect it may be worth creating some template best practices with company formation to increase the likelihood that the businesses retain agency long term in accomplishing AI safety goals, especially given recent events. Aligning business interests with public safety is not just beneficial for societal welfare, but also advantageous for long-term business sustainability—as well as potentially influencing public perception and policy efforts in a dramatically positive way. We also are acutely aware of safety-washing concerns and/or unintentionally creating race dynamics in this domain, and we think that ensuring for-profit safety work is technically rigorous and productive is critical to get right.
- If you are a potential funder for promising businesses that advance alignment, please reach out to us at alignmentangels@ae.studio to express interest in joining our Alignment Angels slack group.
- Scaling our consulting business to do object-level technical alignment work—and then scale this model to many other organizations: the potential to bring other highly promising people into the fold (see point 6, above) to contribute significantly to alignment—even without being alignment experts per se—is a hypothesis we're actively exploring and aiming to validate.
- Given that we expect most people to struggle with having actually-impactful alignment outputs as they are just starting, we see a model where senior AI engineers—even those without explicit alignment backgrounds—can eventually collaborate with a small number of extremely promising alignment researchers who have an abundance of excellent object-level technical project ideas but limited capacity to pursue them. By integrating these researchers into our client engagement framework, used highly successfully over the years for our other technical projects, we could potentially massively scale the efficacy of these researchers, leveraging our team's extensive technical expertise to advance these alignment projects and drive meaningful progress in the field.
- We hope that if this ‘outsource-specific-promising-technical-alignment-projects’ model works, many other teams (corporations, nonprofits, etc.) with technical talent would copy it—especially if grants are made in the future to further enable this approach.
- Neuroscience x mechanistic interpretability: both domains have yielded insights that are mutually elucidating for the shared project of attempting to model how neural data leads to complex cognitive properties. We think it makes a lot of sense to put leading neuroscientists in conversation with mechanistic interpretability researchers in an explicit and systematic way, such that the cutting-edge methods in each discipline can be further leveraged to enhance the other. Of course, we think that this synergy across research domains should be explicitly focused on enhancing safety and interpretability rather than using neuroscience insights to extend AI capabilities.
- Neglected approaches to AI policy—e.g., lobby government to directly fund alignment research: though not a technical research direction, we think that this perspective dovetails nicely with other thinking-outside-the-box alignment approaches that we’ve shared here. It appears as though congresspeople and staffers are taking the alignment problem more seriously than many would have initially predicted and, in particular, are quite open to plausible safety proposals—all of which means that there may be substantial opportunity to capitalize on the vast funding resources at their disposal to dramatically increase the scale and speed at which alignment work is being done. We think it is critical to make sure that this is done effectively and efficiently (e.g., avoiding pork) and for alignment organizations to be practically prepared to manage and utilize significant investment (e.g., 10-1000x) if such funding does in fact come to fruition in the near future. We are currently exploring the possibility of hiring someone with a strong policy background to help facilitate this: while we have received positive feedback on this general idea from those who know significantly more about the policy space than we do, we are very sensitive to the potential for a shortsighted or naive implementation of this to be highly harmful to AI safety policy. We are actively in the process of meeting with and learning more from policy experts: if you are doing work in this area and know way more than us about AI policy, please do reach out so we can learn from you!
We began sharing this ‘Neglected Approaches’ approach publicly at the Foresight Institute’s Whole Brain Emulation Workshop in May, and we were excited to see this strategy gain steam, including Foresight Institute’s own emphasis on neglected approaches with their new Grant for Underexplored Approaches to AI Safety.
We want to make these ideas stronger
It is critical to emphasize again that this list represents our current best guesses on some plausible neglected approaches that we think we are well-equipped to explore further. We fully acknowledge that many of these guesses may be ill-conceived for some reason we haven’t anticipated and are open to critical feedback in order to make our contributions as positively impactful as possible. We intend to keep the community updated with respect to our working models and plans for contributing maximally effectively to alignment. (Please see this feedback form if you’d prefer to share your thoughts on our work anonymously/privately instead of leaving a comment below this post.)
We also recognize that many of these proposals have a double-edged sword quality that requires extremely careful consideration—e.g., building BCI that makes humans more competent could also make bad actors more competent, give AI systems manipulation-conducive information about the processes of our cognition that we don’t even know, and so on. We take these risks very seriously and think that any well-defined alignment agenda must also put forward a convincing plan for avoiding them (with full knowledge of the fact that if they can’t be avoided, they are not viable directions.)
Concluding thoughts
AE Studio's burgeoning excitement about contributing to AI safety research is a calculated response to our updated timelines and optimism about having the skillset required for making impactful contributions. Our approach aims to combine our expertise in software, neuroscience, and data science with ambitious parallel exploration of what we consider to be neglected approaches in AI alignment.
We commit to exploring these directions in a pragmatic, informed, and data-driven manner, emphasizing collaboration and openness within the greater alignment community. We care deeply about contributing to alignment because we want to bring about a maximally agency-increasing future for humanity—and without the precondition of robustly aligned AGI, this future seems otherwise impossible to attain.
We are actively hiring for senior alignment researchers to join/advise our team, in addition to data scientists with a neuroscience background, those highly experienced in policymaker engagement, and a number of other positions. If you have any other questions, comments, or ideas, please do reach out.
[1] Miscellaneous cool accomplishment: before we started getting involved in AI safety in any serious way, two AE engineers with no prior background in alignment developed a framework for studying prompt injection attacks that went on to win Best Paper at the 2022 NeurIPS ML Safety Workshop.
[2] To illustrate this point more precisely, we can consider a highly simplified probabilistic model of the research space. (We recognize this sort of neglect math is likely highly familiar to many EAs, and we don't mean to be pedantic by including it; we've put it here because we think it is a succinct way of demonstrating—if only to ourselves—why taking on multiple neglected approaches is rational.)
Let’s say the total number of plausible alignment agendas is \( n \). Let’s stipulate that currently, alignment researchers have meaningfully explored \( k \) approaches, meaning that \( n-k \) approaches remain unexplored. (As stated previously, we suspect that current mainstream alignment research is likely exploiting only a small subset of the total space of plausible alignment approaches, rendering a large number of alignment strategies either completely or mostly unexplored—i.e., we think that \( n-k \) is large.) Each neglected approach, \( i \), has a very small but nonzero probability \( p_{\text{neglect}_i} \) of being crucial for making significant progress in alignment. Treating these probabilities as independent for the sake of simplicity, the chance that all n−k neglected approaches are not key is \( \prod_{i=1}^{n-k} (1 - p_{\text{neglect}_i}) \). Conversely, the probability that at least one neglected approach is key is \( 1 - \prod_{i=1}^{n-k} (1 - p_{\text{neglect}_i}) \). This implies—at least in our simplified model—that even with low individual probabilities, a sufficiently large number of neglected approaches can collectively hold a high chance of including a crucial solution in expectation. For instance, in a world with 100 neglected approaches and a probability of 99% that each approach is not key (i.e., a 1% likelihood of pushing the needle on alignment), there’s still about a 63% chance that one of these approaches would be crucial; with 1000 approaches and a probability of 99% that each approach is not key, the probability rises to over 99% that one will be pivotal. This simple model motivates us to think it makes sense to take many shots on goal, pursuing as many plausible neglected alignment agendas as possible.
[3] Please note: (1) we are primarily interested in aggregating the best ideas to begin, so don’t worry if you have an idea that you think fits the criteria above but is challenging to implement/you wouldn’t want to actually implement it. (2) There is space on the form to denote that your suggested approach is exfohazardous.
[4] This is a core trade-off in our work and something that we have made substantial progress on since our founding.
[5] We want to call out that this approach is likely the least neglected of the ten we enumerate here—which is not to say it isn’t neglected in an absolute sense.
[6] While there are a good number of newer organizations working on fieldbuilding for alignment, we think it remains highly neglected given the potential impact, especially in likely-impactful fields that are only now starting to be considered within the Overton window.